To engage in social science research is to contend with imperfect information, uncertainty and human error. Conducting research on international development finance is no exception; even the data that donor institutions themselves vet and publish contain errors, biases, and inconsistencies. Social scientists have learned that it is far easier to deal with imperfect information when strict standards of transparency are upheld. Errors can be more easily identified and corrected. Biases can be more easily expunged. And uncertainty can be explicitly modeled or otherwise addressed.
AidData has embraced the notion that ‘transparency is the best disinfectant’ and prioritized the timely disclosure of its data and the methods used to generate the data. To this end, AidData’s Tracking Underreported Financial Flows (TUFF) team has decided to provide users of its data products with user-friendly measures of data accuracy and completeness. When our team first published the 1.0 version of our Chinese Official Finance to Africa dataset in April 2013, we also released an extensive codebook documenting each step of our data collection and quality assurance process. However, since that time, it has become clear that many of our users would like simple and intuitive summary metrics of data quality at the project-level.
Introducing the “Health of Record”
In response to these concerns, our team has developed a scoring system that we call our “Health of Record” methodology. It rates each project on two dimensions: a source triangulation score that captures the number and diversity of information sources supporting a given project record, and a data completeness score that measure the extent to which project fields/variables are populated with missing or vague information..
The source triangulation score varies from 0 to 20, and is calculated by awarding points for each media report, government source, or academic article that informed its creation. Since official reports and academic articles are generally more reliable than media reports, we assign a higher weight to these information sources (see Appendix N). A project also receives “bonus points” if we have verified its existence via in-country data collection. The data completeness score varies from 0 to 9, with higher scores indicating project records that benefits from more complete information. It deducts points when a record is missing or has vague information for key fields (see Appendix N).

Figure 1: Source Trangulation Scores
These scores have many potential applications, and one use is to track data quality changes over time. Figures 1 and 3 show the change in ‘health of record’ scores across various iterations of AidData’s Chinese Official Finance to Africa dataset: first, the 1.0 version released in April 2013; then, the 1.1 version released in January 2014; and finally, the 1.2 version released in October 2015.

Figure 2: Distribution of Information Sources by Dataset Version
The source triangulation scores reveal both an increase in the number and diversity of sources being used. In the 1.0 version of the Chinese Official Finance to Africa dataset, over 60% of project records were informed by only one or two media reports. With the release of the 1.2 research release, we can see that this number dropped sharply -- to just over 30%. In Figure 2, we can also see that diversification of the information sources used to inform each project record has led to a major improvement in the topline (as opposed to project-level) source triangulation score. These improvements are largely the result of our team’s decision to adopt a new set of rigorous rules about how to classify Chinese official finance projects.
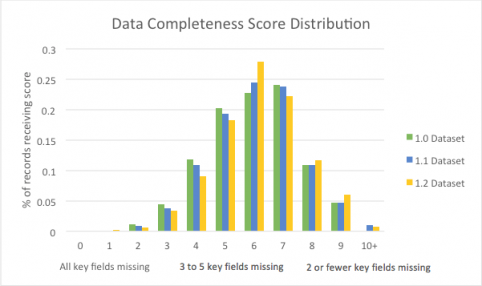
Figure 3: Data Completeness Score Distribution
In the coming months, AidData will post these ‘health of record’ scores on the individual project pages of the china.aiddata.org portal to give users a more dynamic view of how the quality of the database is changing over time. We hope that the publication of these scores will spur a conversation about how best to capture and characterize data quality at the project level.