Leveraging machine learning algorithms to sift through terabytes of high-resolution satellite data, a new report by AidData and the Global Environment Facility has identified the factors that contribute to land degradation on a global scale.
Land degradation occurs when the environment is negatively affected by human activity, resulting in the loss of agricultural productivity, forest cover, biomass, or biodiversity. A growing threat, it is estimated that up to 40% of the world’s agricultural lands are seriously degraded.
The Global Environment Facility (GEF) has worked for years to combat land degradation in forests around the world, and needed to understand where they were succeeding — and why. To find out, AidData and the Independent Evaluation Office of the GEF joined together to assess the impact of GEF land degradation projects using cutting-edge research methods.
To measure project impact, AidData researchers used machine learning algorithms, a type of artificial intelligence (AI) that provides computers with the ability to learn without being explicitly programmed. These computer programs teach themselves to grow and change when exposed to new data.
Report author and AidData geospatial scientist Dan Runfola explained the study’s unique methods: “We trained our algorithm to sort through higher fidelity satellite data [30 meter resolution] than the usual 500 meter resolution common in global-scale studies. This meant that we could see changes to individual trees, not just the whole forest. Our algorithm was then able to compare areas with GEF projects to similar areas without those projects, at a very fine level, and build an accurate counterfactual of what would have happened to the land, good or bad, without those projects there.”
Across the globe, GEF projects were found to increase both the number of trees in forests and the number of leaves on those trees. A value-for-money assessment shows that the average GEF project, which costs $4.2 million USD, sequesters around $7.5 million worth of carbon. The initial condition of the land where a project was located and its distance from urban areas both turned out to be key predictors of impact: projects tended to have a larger impact in areas that were more degraded to begin with, while projects located closer to urban areas tended to be less effective.Integrating research methods from three very different disciplines (geospatial analysis, econometric modeling, and artificial intelligence), this study is the first of its kind to use machine learning to not only sift through terabytes of satellite data and explicitly tie projects combating land degradation to positive changes in the environment, but also to mine the data to find insights for future program design.
Value of GEF land degradation projects
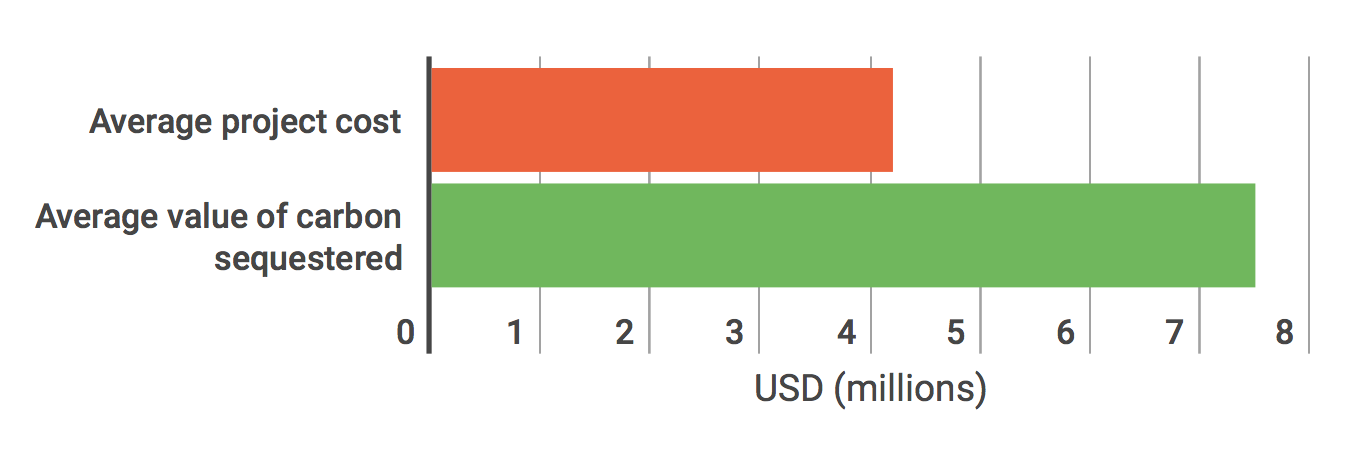
Integrating research methods from three very different disciplines (geospatial analysis, econometric modeling, and artificial intelligence), this study is the first of its kind to use machine learning to not only sift through terabytes of satellite data and explicitly tie projects combating land degradation to positive changes in the environment, but also to mine the data to find insights for future program design.
“Building data models based on real world scenarios and then mining them for spatial heterogeneity — which means, the differences in data points within a specific location — helps us find insights that may seem intuitive in hindsight, but that we otherwise never could have seen,” notes Runfola.
“For example, we observed a lag of about five years between when the programs to combat land degradation were implemented, and when they started having impact. Intuitively, that makes perfect sense: trees take time to grow, and maybe that time is about five years. This is a completely data-driven finding that helps the GEF plan for when they should begin future impact evaluations of their projects.”
Another discovery that will help the GEF moving forward is that projects in Asia and Africa were not successful in mitigating forest fragmentation, resulting in smaller, less contiguous forests than projects in other areas. The report notes that the initial state of forest fragmentation remains a key determinant in the average size of a forest tract.
“The big idea here is global-scale positive deviance from the norm,” says Runfola. “What this geospatial impact evaluation methodology does is point us toward where to look for the needle in a haystack of thousands of variables. If you visit http://labs.aiddata.org/gef and view the global map of GEF projects, you see a group of unsuccessful projects in Sub-Sarahan Africa. But amid this cluster of red dots, your eye is immediately drawn to that one green dot, that one successful project. And you can zero in on it to ask: what made this project successful where everything else failed? The machine learning algorithms we used in this study sort through the data, and learn to identify and pinpoint these so-called 'bright spots,' these areas of positive deviance — without being told how and where look for them. Now, evaluators can see clearly and instantly which areas merit further investigation.”
Moving forward, AidData will conduct three additional rounds of analysis with the GEF, and contribute to the 6th evaluation of the GEF by the Independent Evaluation Office, as mandated by the UN. AidData will also examine how project design and funding type contribute to GEF project success, and investigate the impact of the GEF’s portfolio on biodiversity outcomes.