Machine Learning
Overcoming limited data through next generation outcome measures
Case Studies
Ghana
Improving the sophistication of poverty estimates
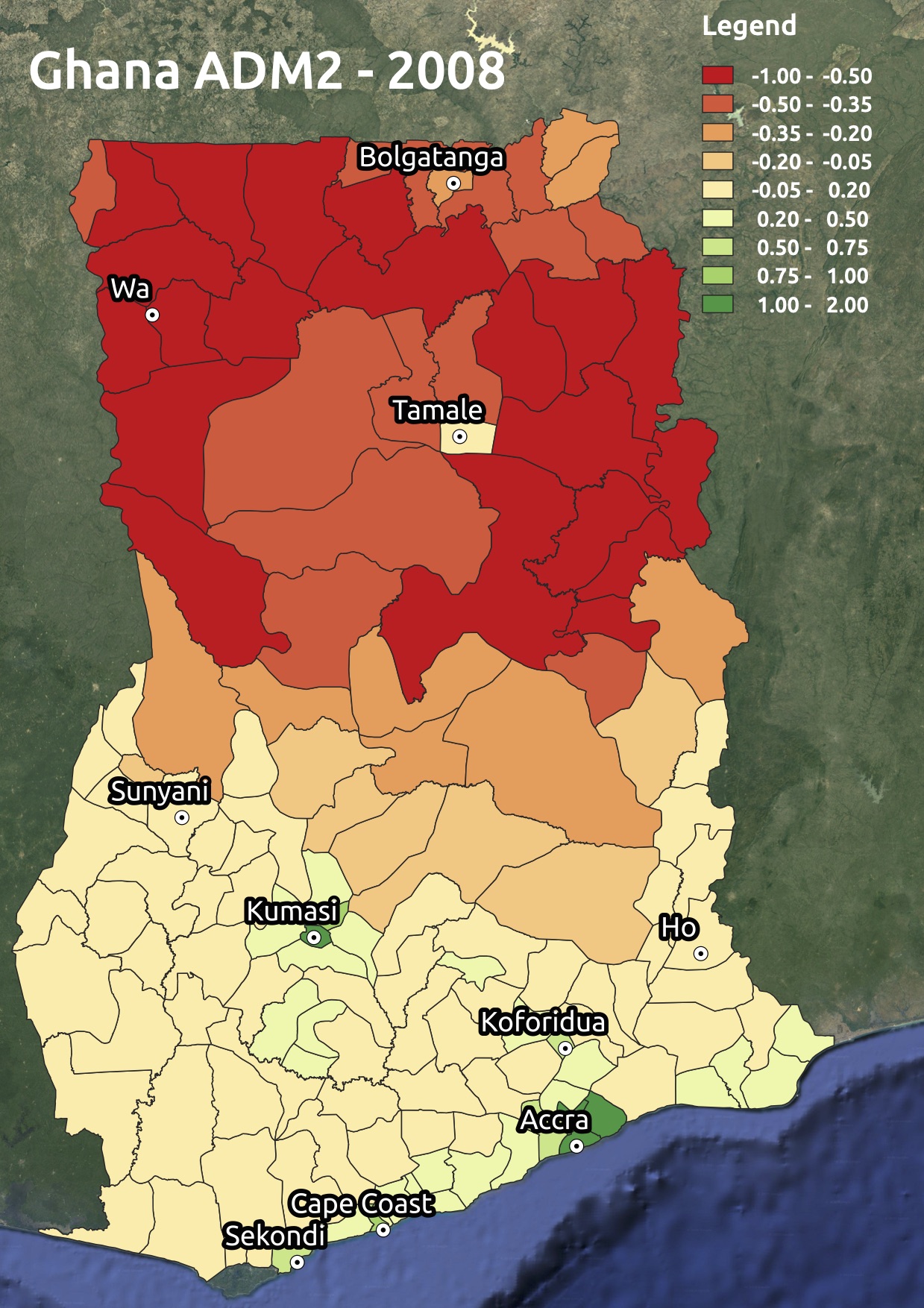
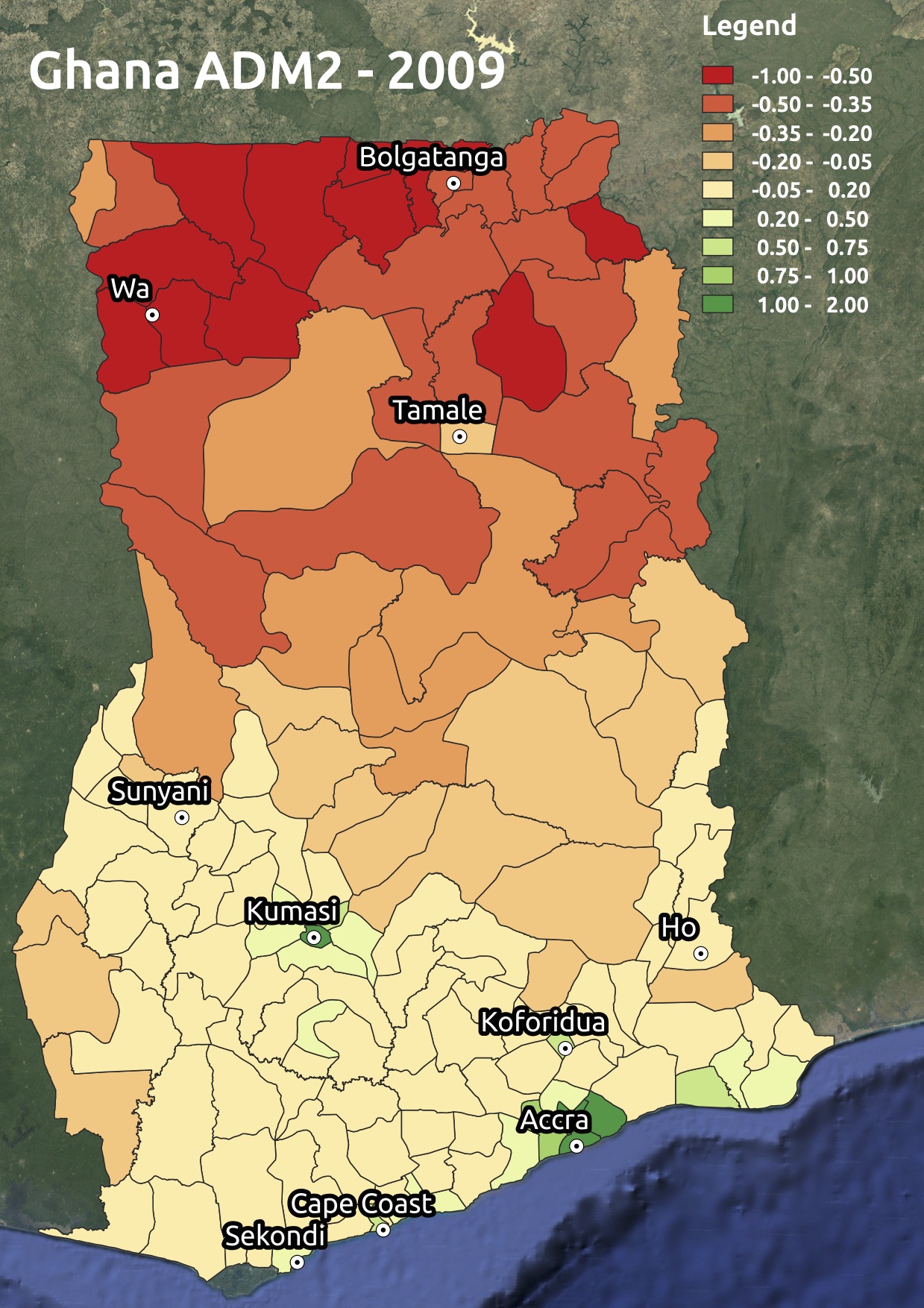
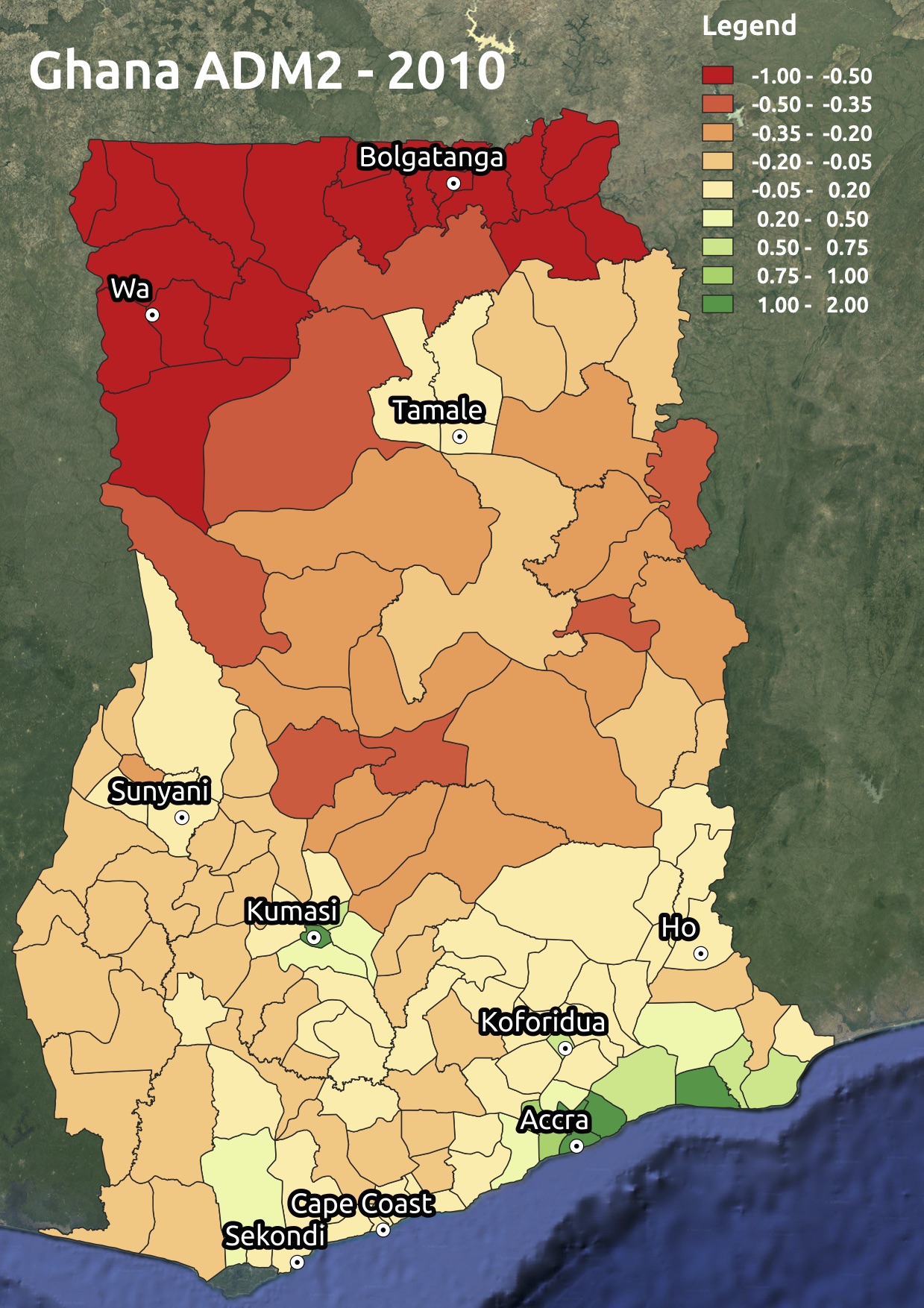
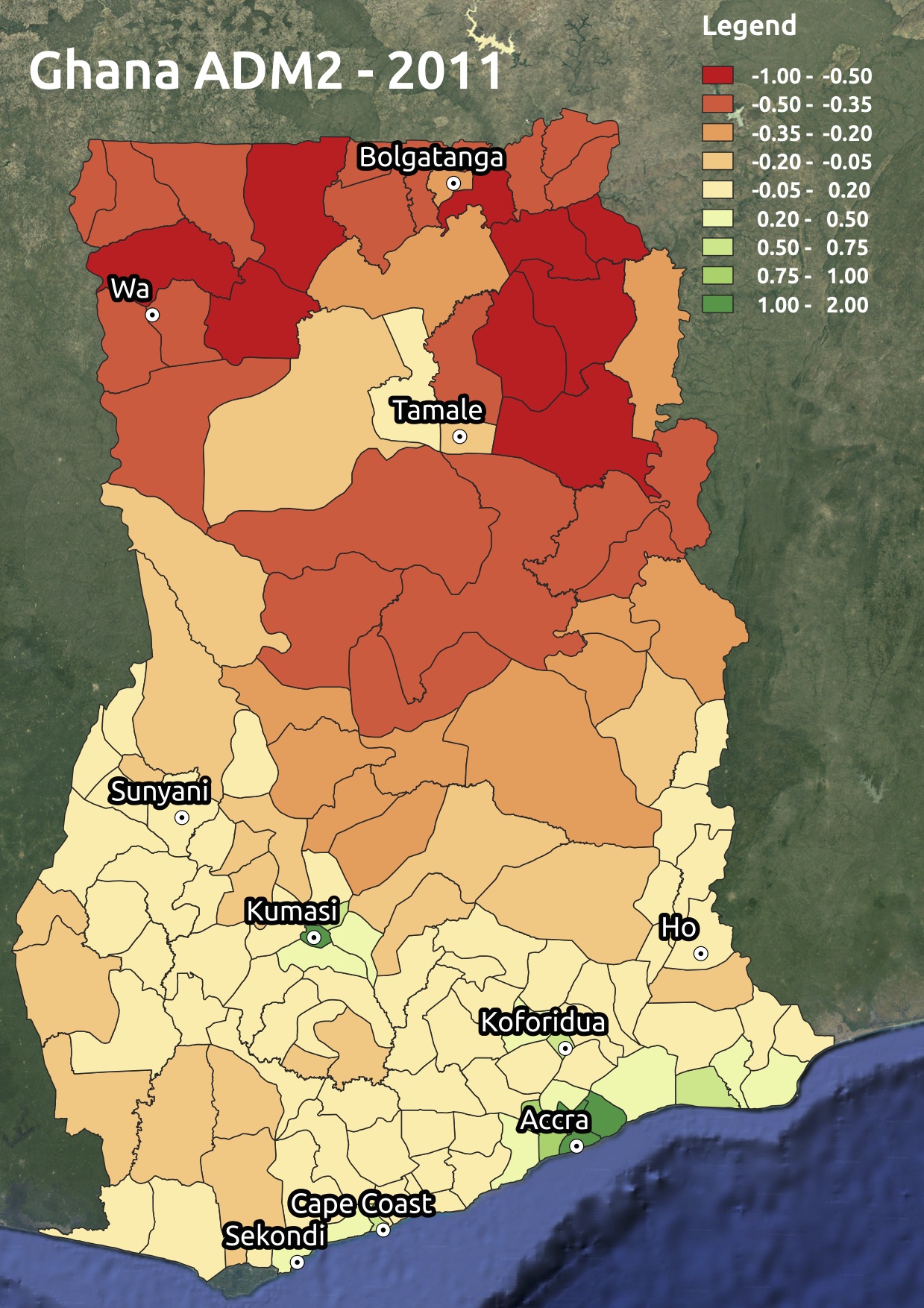
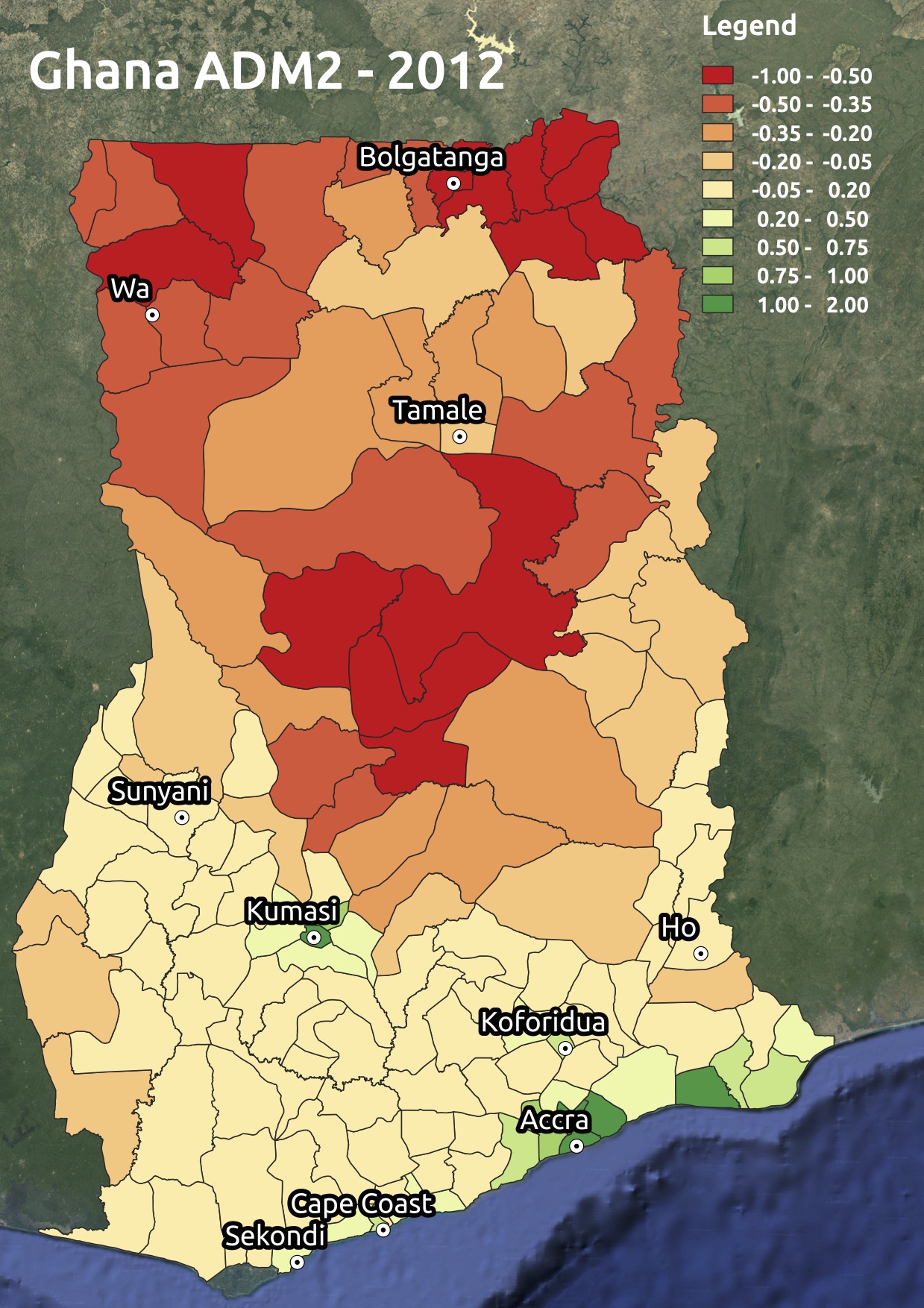

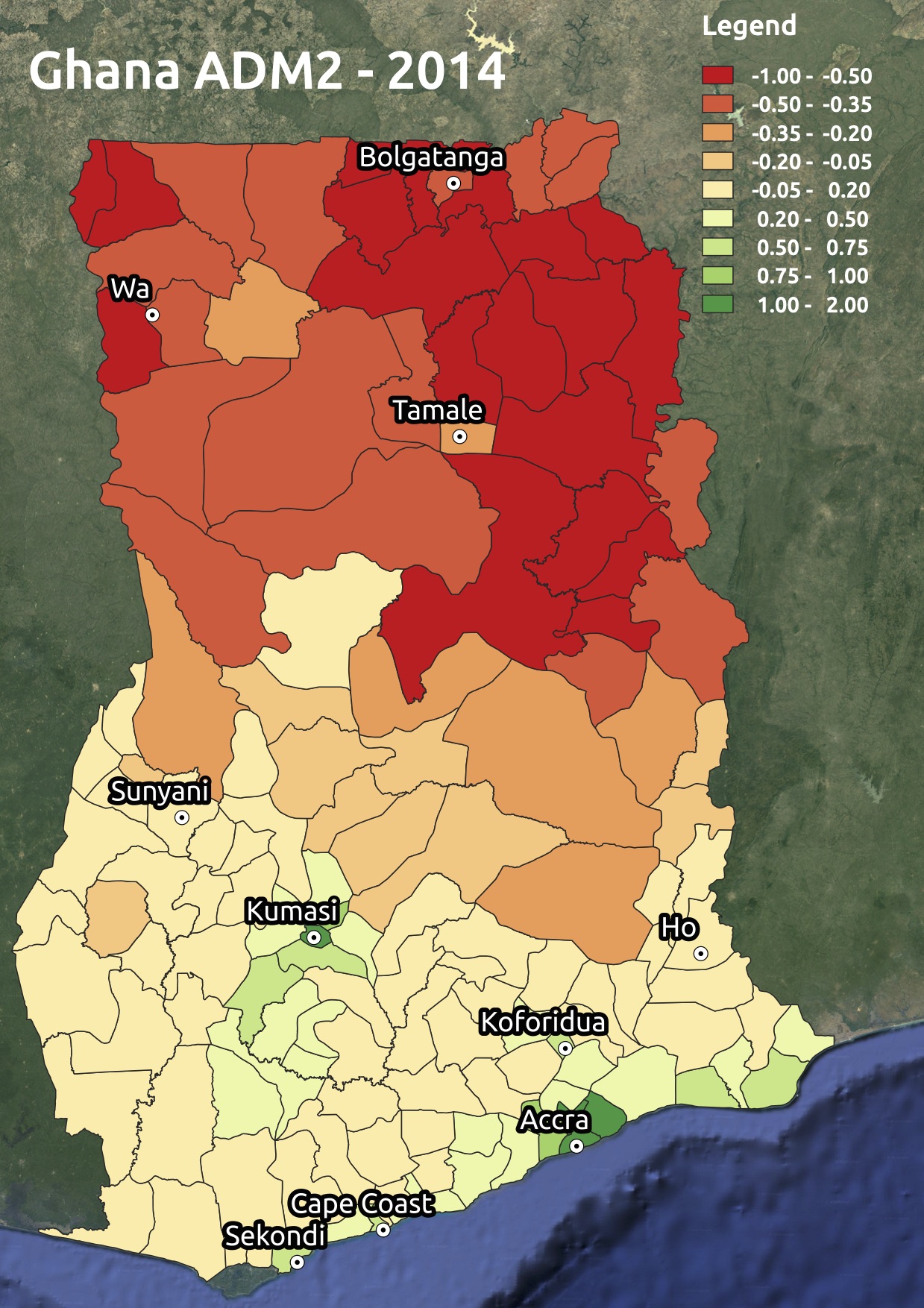
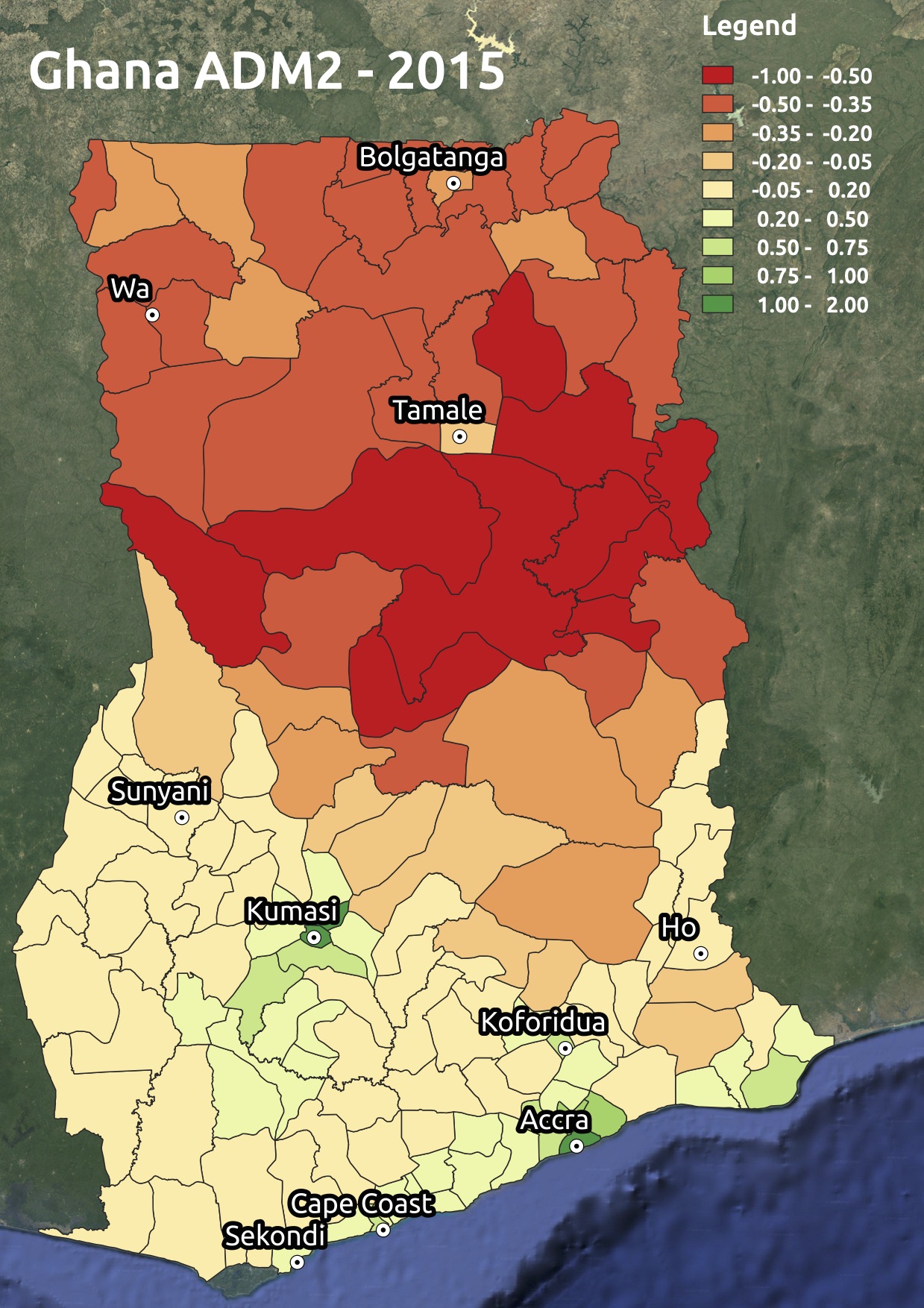
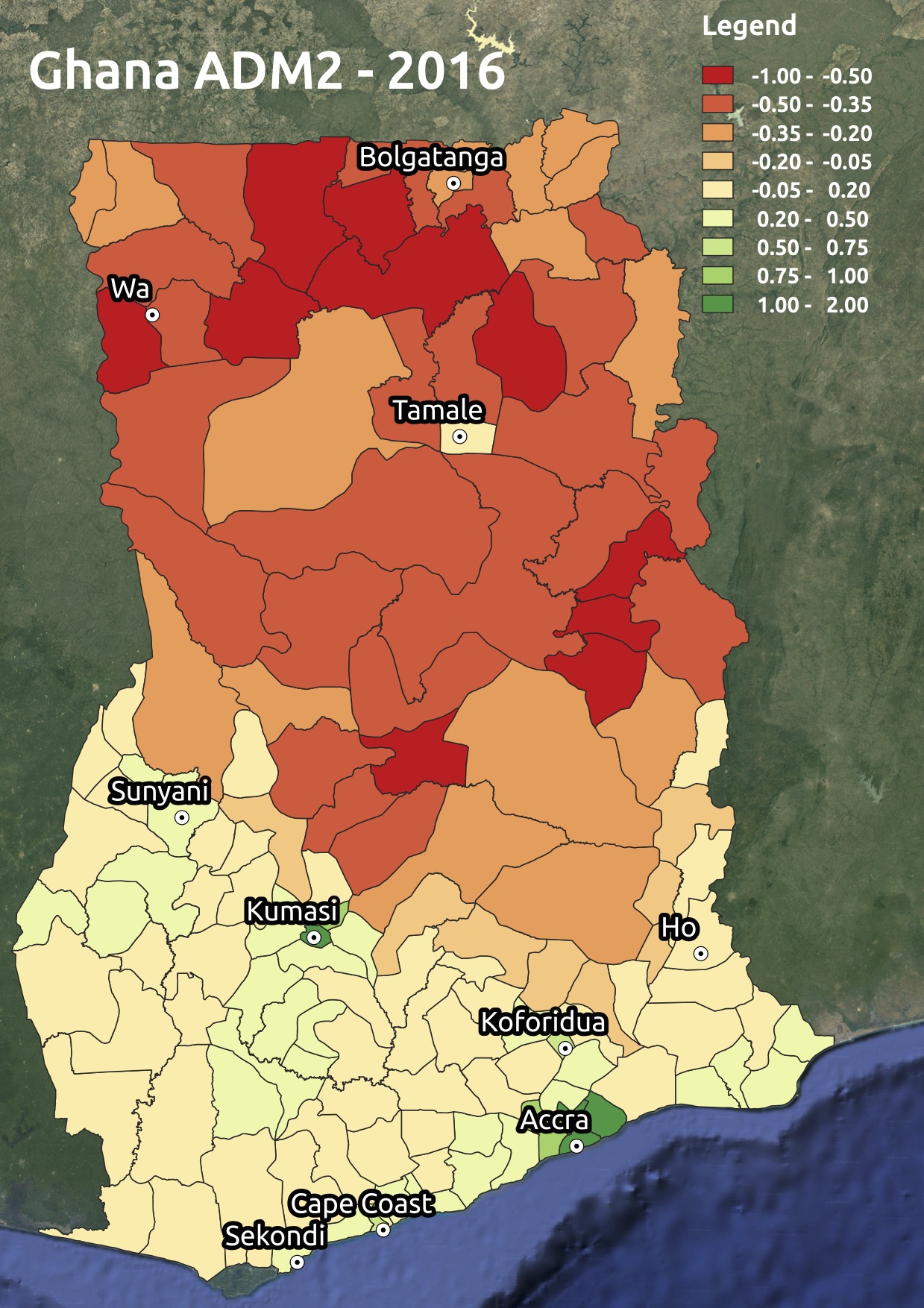
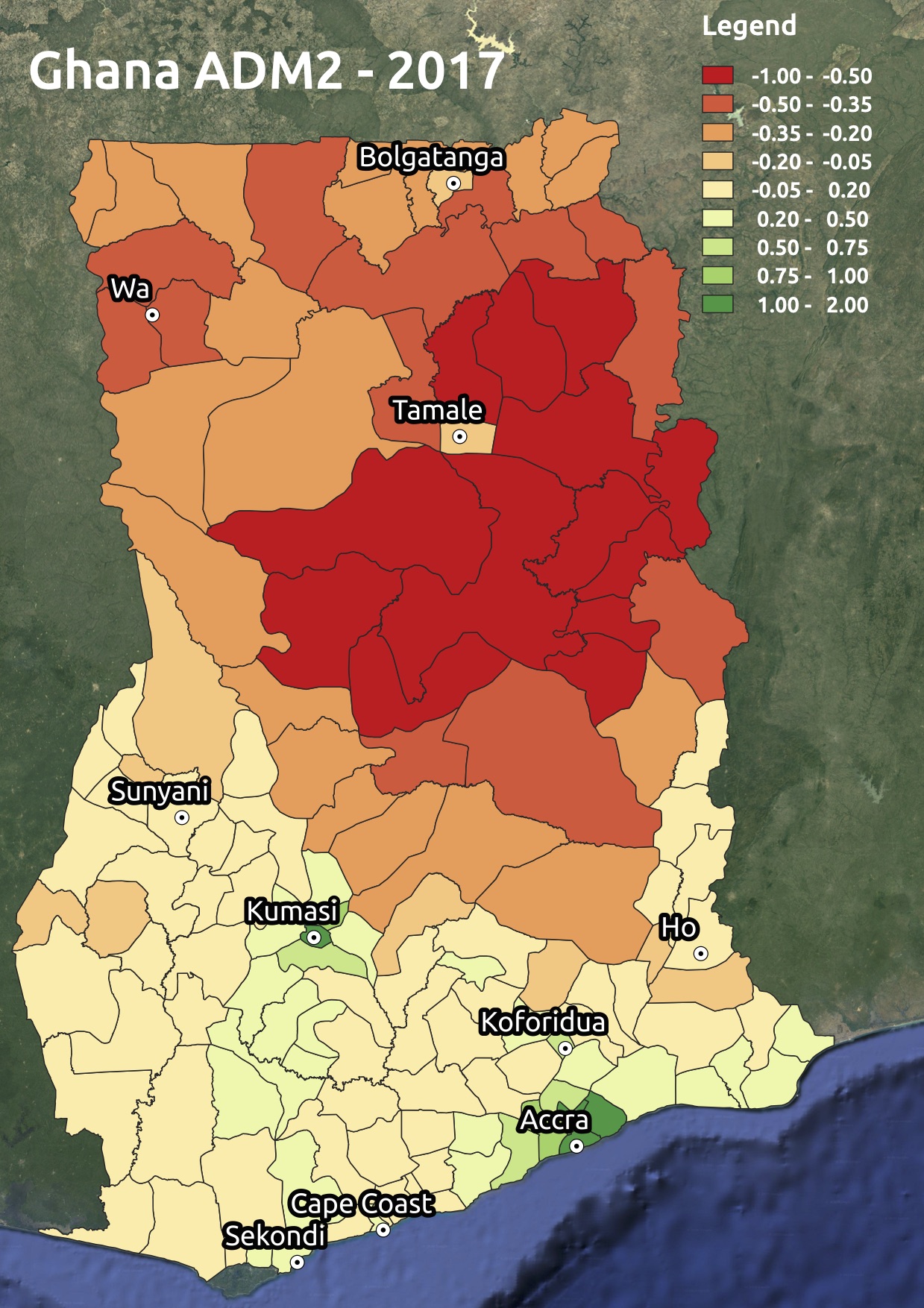
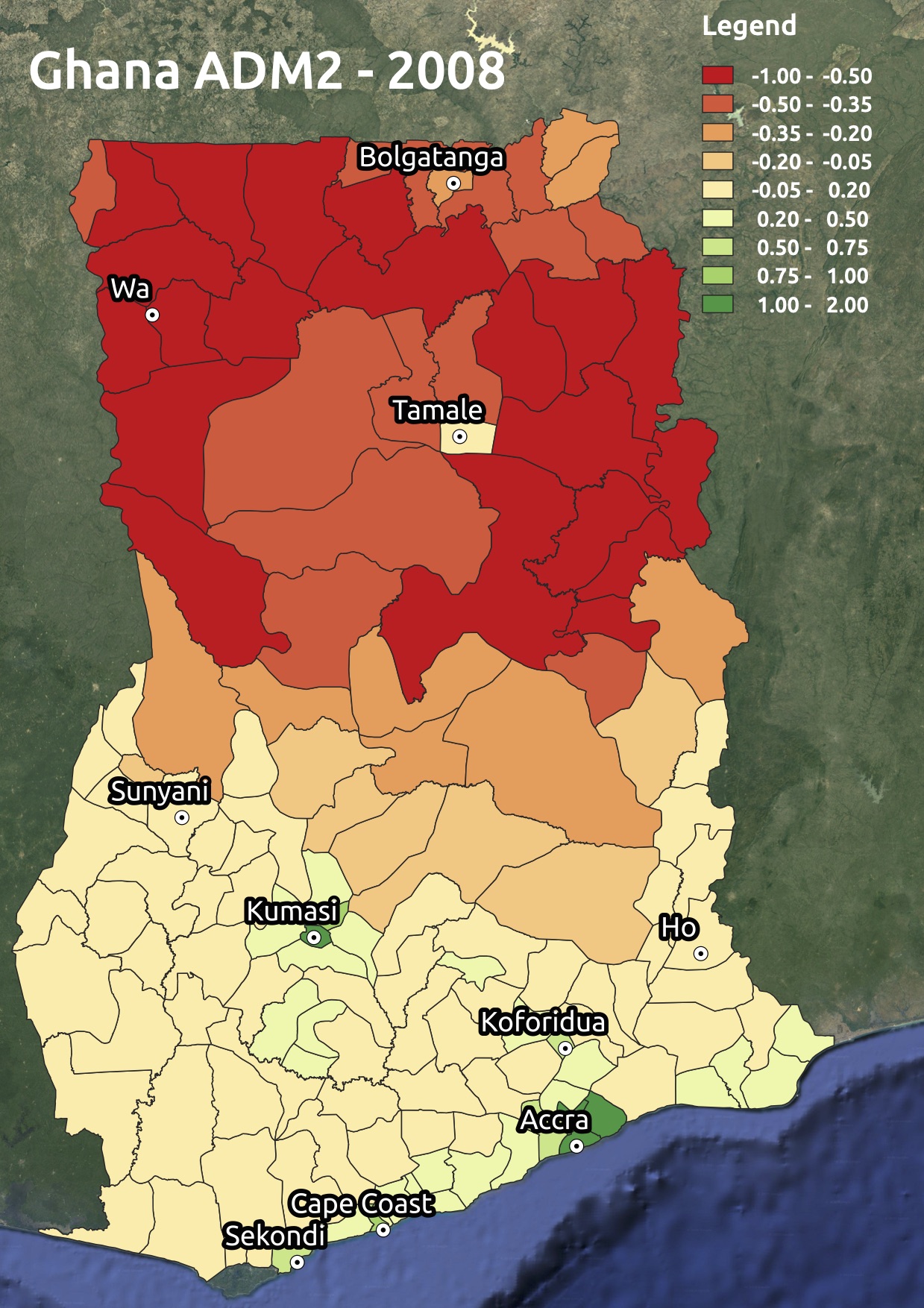
Case Study
All data sources have limitations. When it comes to measuring poverty, surveys are expensive, limited to small sample sizes, and available only for certain geographic points in certain years. Proxies for poverty such as nighttime lights are imperfect and often struggle to accurately measure populations most in need. AidData is expanding upon methods pioneered by Stanford researchers to overcome these challenges and produce next-gen measures of poverty by leveraging machine learning.
To better estimate the economic impact of improved market access from an MCC program in Ghana, AidData utilized DHS surveys and Landsat 7 satellite data along with convolutional neural networks to provides more accurate estimates of changes in average household wealth. The resulting estimates were used to compare changes in poverty over time between regions around road improvement projects and control locations that were not near improved roads.
Download the data (beta)
Nigeria
Providing a low-cost solution to predict the likelihood of deadly conflict
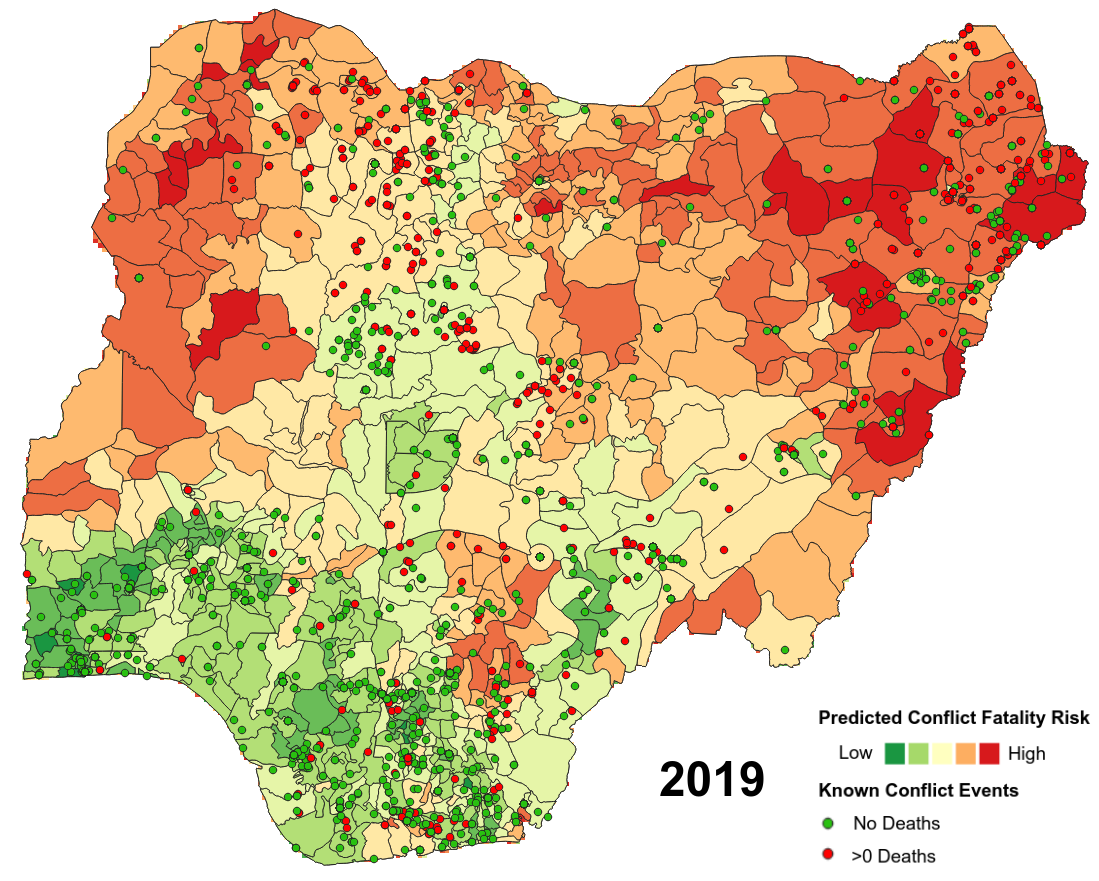
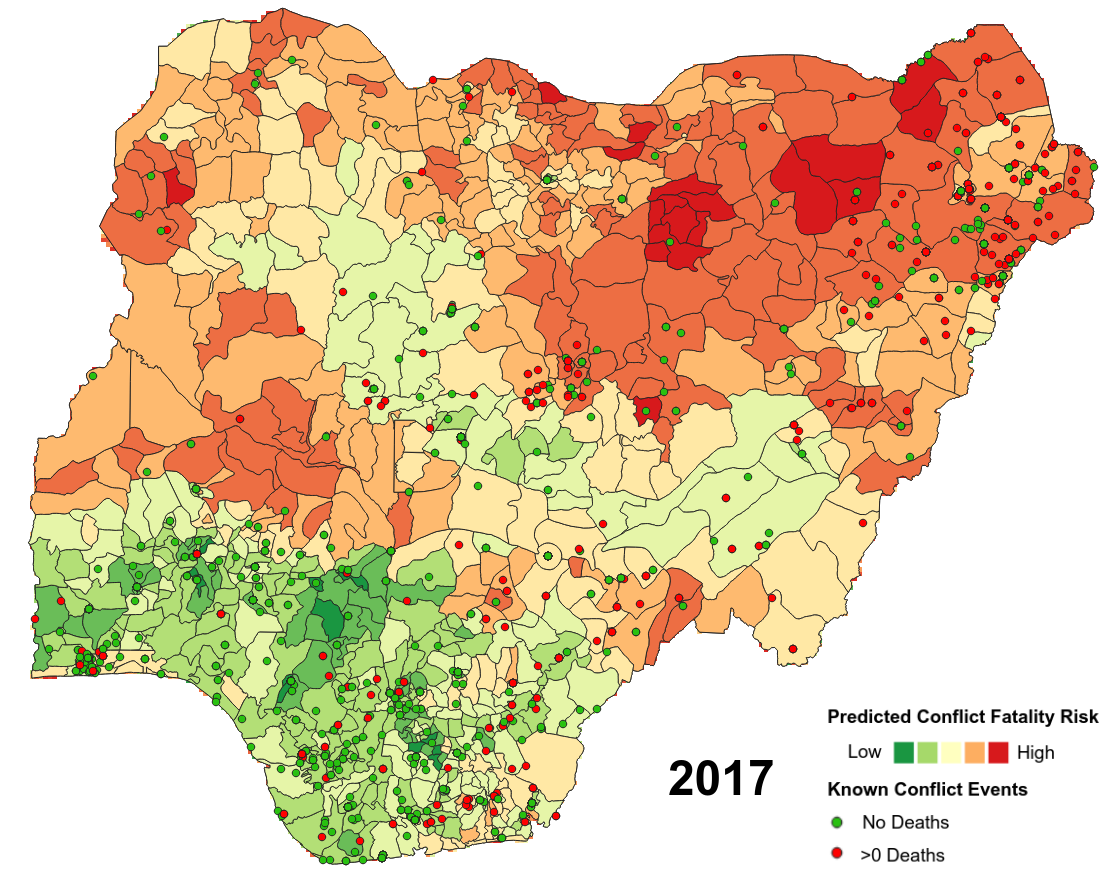

Case Study
Uncertainty limits the humanitarian response in areas with known conflicts. AidData is working to improve how existing data can be used to train convolutional neural networks that make more accurate predictions of the likelihood of a death due to conflict at a given location. To make these estimates, the algorithm analyzes landscape features detected from moderate-resolution satellite imagery the previous year. Using Nigeria as a case study, this algorithm achieves approximately 80% classification accuracy when predicting whether a location with a known conflict in 2015, 2017, 2019 was fatal based on previous year's satellite imagery, at either yearly or six month intervals.
Read the research
Download the data and technical report
License
Data provided on this page falls under the terms of AidData's GeoQuery license. Please read the GeoQuery license before using this data. Specifically, please note the terms in the disclaimer section. This includes the following. Data are not to be considered as advice or guidance on issues or activities related to its content. Data are provided "as is" without any representations or warranties as to their accuracy, completeness, performance, merchantability, or fitness for a particular purpose. The entire risk associated with the results and performance of the data is assumed by the user.