Development practitioners, governments, and local stakeholders face a fundamental challenge: many areas, precisely where development investments are most needed, lack reliable data on poverty levels and household wealth. These areas may be too remote or unstable, or national and local governments may lack the capacity to field regular censuses. How, then, can policy makers and international development organizations allocate resources to the areas with the greatest need? Or, just as importantly, measure whether programs are having the intended impacts?
Increasingly, wealth estimates—generated using artificial intelligence (AI) trained on the survey data on household wealth that we do have—are being used to fill the gap. Enabled by improvements in modern deep learning AI models—such as convolutional neural networks that can analyze large amounts of satellite imagery and other geospatial data more cheaply and quickly than ever before—AI-generated wealth estimates are being rapidly adopted for development research, evaluation, and decision-making applications.
Read the full report, Evaluating Gender Bias in AI Applications Using Household Survey Data
But a major unknown is whether and to what extent these estimates have gender bias baked into them from the beginning. The Demographic and Health Surveys (DHS) Wealth Index (WI), produced by USAID, is one of the most widely used sources of training data for AI models that estimate wealth. Numerous studies have found that AI-based models perform well at estimating wealth on average. Yet until now, no research has explored how well they accurately capture women’s wealth specifically. As AidData’s newest research initiative on Gender Equity in Development has found, development programs can have different impacts on men and women that household surveys often do not capture or incorrectly measure. Designed properly, AI models that draw on this data could help detect and minimize gender bias—or, if designed poorly, exacerbate it.
Recognizing that AI technologies could have not only extraordinary benefits but also the strong potential for gender-biased impacts, USAID launched the Equitable AI Challenge. The Challenge was designed to support approaches that increase the accountability and transparency of AI systems in global development contexts. Last fall, AidData and CDD-Ghana, a research and advocacy think tank, were announced as one of five winners of the Challenge. Our partnership has worked over the past year to evaluate the potential impact of gender bias on wealth estimates generated using AI and USAID’s DHS data, in order to inform AI developers and researchers, development organizations, and decision makers who produce or use wealth estimates.
Over the course of the project, AidData led the technical development and analysis of the machine learning models used to estimate wealth. Our research team has created a practical and extendable methodology for evaluating the potential gender bias of those models. CDD-Ghana incorporated local, contextual understanding to inform the development of the machine learning models and engaged with in-country stakeholders and organizations. The involvement of a local partner such as CDD-Ghana is increasingly critical in AI applications, as it helps shape how models are built and trained and adds key context to the results. The importance of local knowledge also highlights the limits of AI-based wealth estimates, as it may not always be possible to draw generalized predictions if local conditions vary significantly. The results are available in a report released today, and the related code and data have been published in the public domain.
According to the report’s executive summary, “the lack of previous research into the role of gender in AI-based wealth estimates, combined with unique challenges of the data used, meant that the scope of work was ambitious…Many established approaches for considering gender bias in AI training data, or in trained models themselves, could not be directly applied.” To overcome these challenges, the researchers took an approach that involved classifying households surveyed in the 2014 Demographic and Health Survey (DHS) in Ghana by gender, differentiating between male- and female-headed households, as well as other household conditions identified by CDD-Ghana. They then trained separate Gender-specific Random Forests (a type of AI model) on the gender-classified DHS data, in order to compare the models and understand which ones performed best at predicting household wealth.
The AidData-CDD research team found that AI models trained and validated on male household data outperformed models trained and validated on female data at predicting household wealth—suggesting that gender is meaningfully influencing the performance of the models.
The in-country aspect of our year-long collaboration culminated last month in a dissemination workshop hosted by CDD-Ghana at their headquarters in Accra, Ghana. Over 40 in-person and two dozen virtual attendees participated in an engaging discussion on “Evaluating Gender Bias in AI Applications,” with panelists who are experts in AI, gender, and population statistics. These included Selaseh Pashur Akaho, a statistician from the Ghana Statistical Service (GSS); Dr. Rita Udor, a gender inclusivity officer from the Responsible AI Lab at the Kwame Nkrumah University of Science and Technology (KNUST); and Deborah Dormah Kanubala, a machine learning researcher at Saarland University in Germany.

A wide diversity of attendees shared their perspectives on the role of equitable AI and wealth estimates in Ghana; the broader context of gender bias; the future of AI; and the importance of ongoing efforts to ensure minority groups are not harmed by rapidly advancing technologies. Rachel Sayers, an AidData Research Scientist and one of the principal investigators on the Equitable AI project, presented the group’s preliminary findings on evaluating gender bias in AI applications using household survey data. A recording of the workshop is available below.
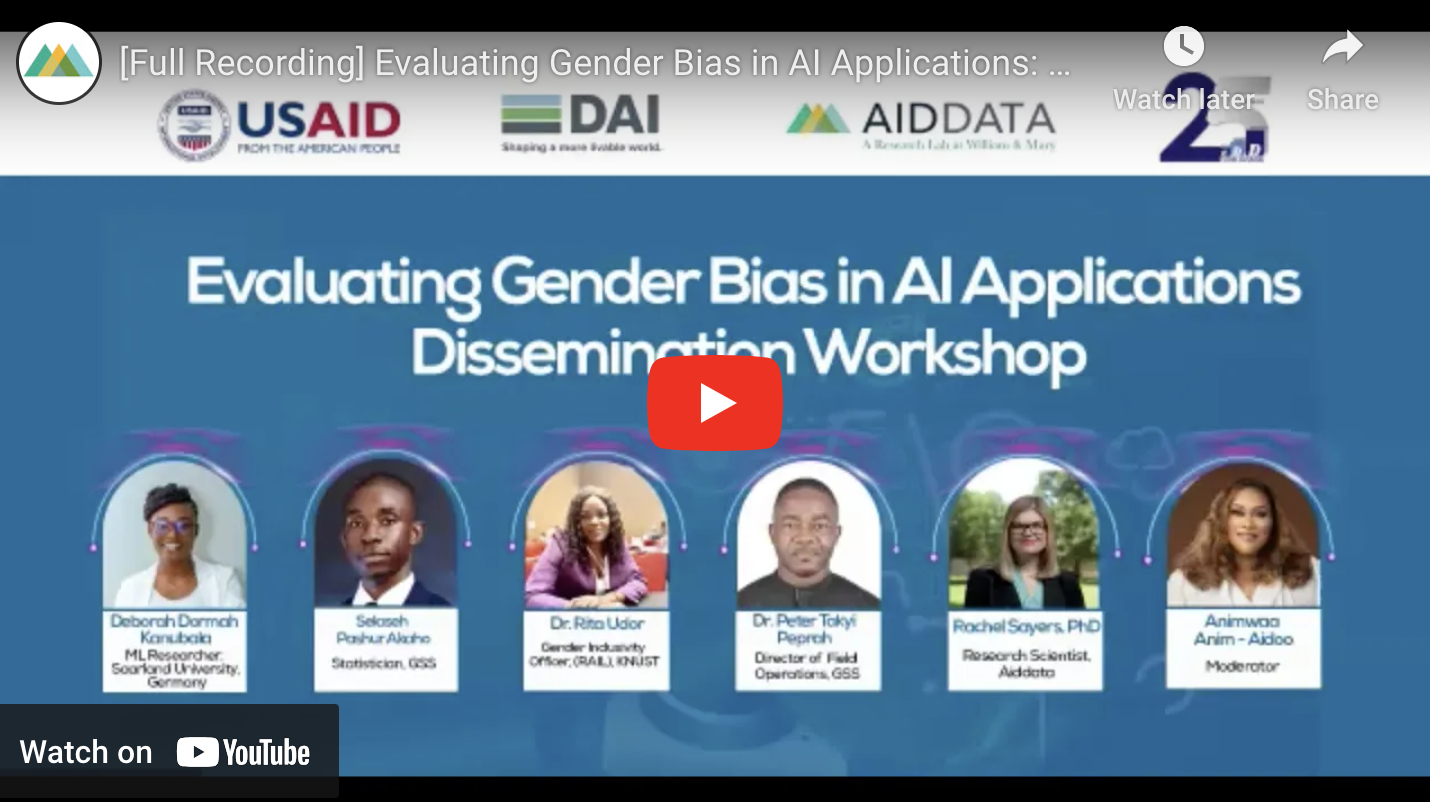
Nine local media organizations in Ghana also attended the workshop, resulting in stories by the Ghana News Agency, All Africa, and Graphic Online, plus coverage on Ghana Broadcasting Company (GBC) Radio. Sayers and Mavis Zupork Dome, a Research Analyst at CDD-Ghana, were interviewed the next day on GBC’s Uniiq FM Breakfast Drive, reaching a wide local audience with a public interest story on the promises and pitfalls of AI with regards to gender.
Going forward, AidData plans to build on this work by making our own uses of AI-based wealth estimates in geospatial impact evaluations more gender-equitable. The researchers involved—Sayers; Seth Goodman, a Research Scientist; and Katherine Nolan, a Research Scientist and co-leader of the Gender Equity in Development Initiative—also plan to produce an academic publication based on this project, to disseminate their findings to fellow researchers.