In partnership with CDD-Ghana, AidData has worked to evaluate the potential of gender bias in wealth estimates generated using artificial intelligence (AI), geospatial data, and USAID’s Demographic and Health Surveys (DHS) data. The project leverages AidData’s expertise in artificial intelligence, geospatial data, household surveys, and CDD-Ghana’s knowledge of the local context and environments to produce a novel public good that will elevate equitability discussions surrounding the growing use of AI in development. Funded through USAID’s Equitable AI Challenge (and implemented through DAI’s Digital Frontiers agreement with USAID) this project approaches a critical problem: increase the accountability and transparency of AI systems used in global development contexts. The project builds upon AidData’s broader research initiative on gender equity in development, as well as ongoing AI applications.
Ghana Workshop
On August 16 at the Ghana Center For Democratic Development (CDD-Ghana) headquarters in Accra, AidData and CDD-Ghana conducted a workshop to share findings from our research and discuss gender bias in AI applications for global development. AidData Research Scientist Dr. Rachel Sayers was joined by experts from CDD-Ghana, Ghana Statistical Service, and the Kwame Nkrumah University of Science and Technology's Responsible AI Lab (KNUST RAIL), and Saarland University for an engaging discussion.
Community of Practice
The Equitable AI Community of Practice - facilitated by USAID, DAI, and Atlantic Council as part of the Equitable AI Challenge - aims to bring together individuals and organizations interested in learning and working towards a more equitable AI-powered future.
Join the Community of Practice Group on LinkedIn
Access the data and code
We utilize random forests regressions (RFRs) and features derived from a range of geospatial datasets (nighttime lights, land cover, OpenStreetMap features, and more) to produce estimates of household wealth. All code and the majority of the data have been made directly available through our GitHub repository, and includes documentation for replication.
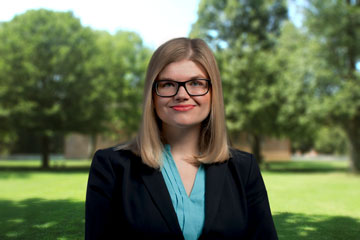
Rachel Sayers
Research Scientist
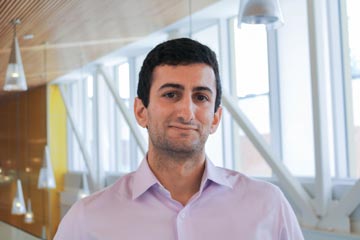
Seth Goodman
Research Scientist
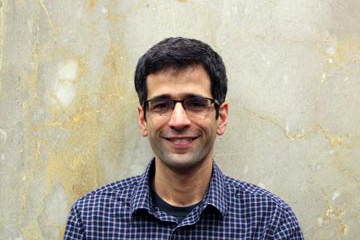
Ariel BenYishay
Chief Economist, Director of Research and Evaluation
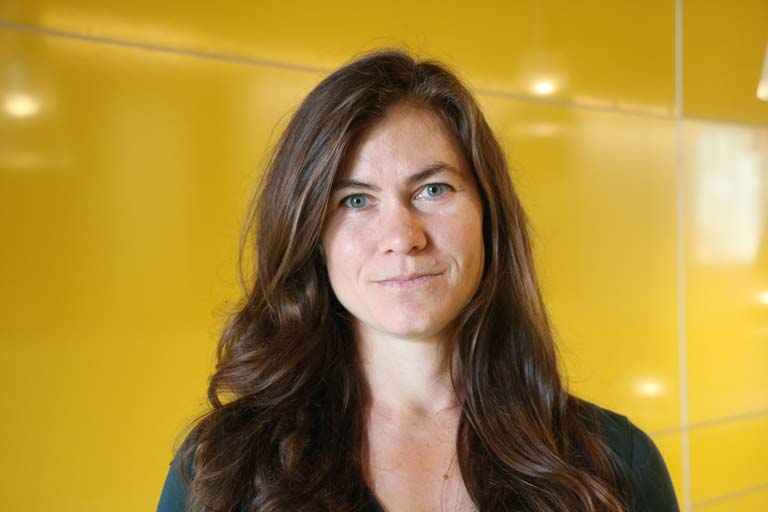
Katherine Nolan
Research Scientist
For partnerships and media queries, contact:
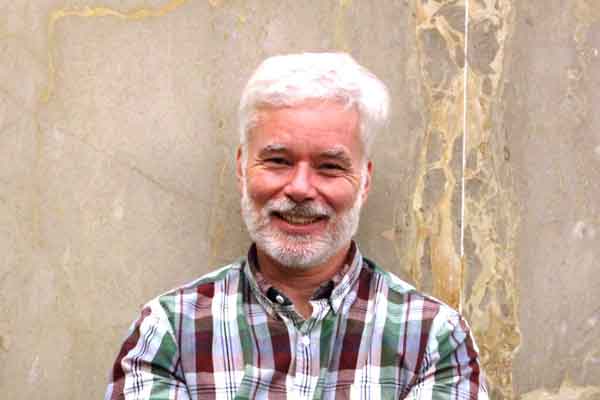
Alex Wooley
Director of Partnerships and Communications
Approach
The DHS Wealth Index, an asset-based metric of household wealth, is one of the most widely used sources of training data for AI models which estimate wealth. AI-based wealth estimation models have been shown to perform well in general across numerous studies, yet no work has explored their effectiveness at accurately accounting for conditions for subpopulations, such as women. To explore variation in model accuracy for households led by women or men, we classify households surveyed in the 2014 Ghana DHS by gender and train separate models in order to compare them.
Since DHS assets are only recorded for the entire household, accounting for gender-specific conditions can be difficult. We classify households as male or female using varying methods including the gender of the head of household, as well as using country-specific context and trends related to gendered asset ownership and control identified by CDD-Ghana in a detailed report. Gender-specific AI models are trained using the Wealth Index as the dependent variable, and a range of geospatial data from satellite imagery and other sources as the independent variable.
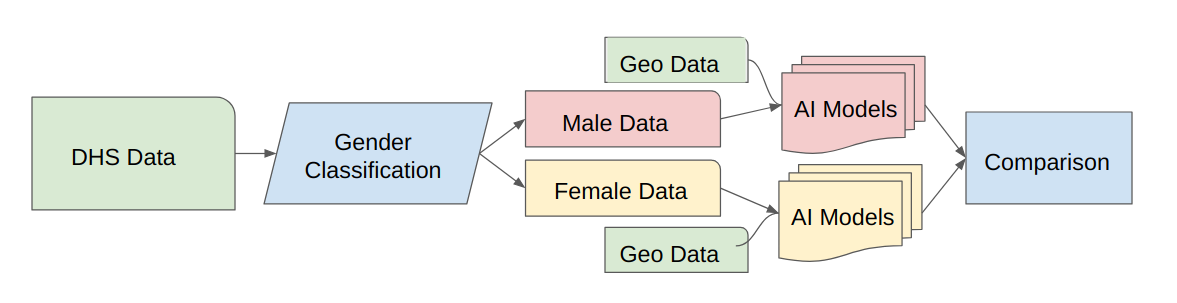
The geospatial data (including nighttime lights, population, land cover, and more), along with the code used to train models, are publicly available to support replication and future use.
Findings
We test the performance of the AI models trained across a range of parameters and inputs that impact model behavior, using data for each gender. Across tests, models trained on male household data consistently outperform - or more accurately estimate wealth - models trained on female household data.
A critical factor is the differences in the number of male and female households used to train the models. As there are typically more male households, we run robustness tests in which we limit the number of households to be equal across genders. We find that when using equal household counts, male models still outperform female models, suggesting gender is still meaningfully influencing model performance.
A valuable feature of the type of AI models used is the ability to measure the importance of specific features (the geospatial variables) used to estimate wealth in the models. Male and female models had similar feature importance overall, and were most heavily influenced by a subset of features, including nighttime lights, urban area coverage, and population. Notable differences include male models being more influenced by rainfall amount, while female models were influenced by distance to cities.
Additional efforts to understand the influence of gender on the DHS Wealth Index itself involved rebuilding the index using gender-specific data. We found that the gender-specific wealth index created for female-led households tended to classify poorer households as even poorer relative to the original DHS WI.
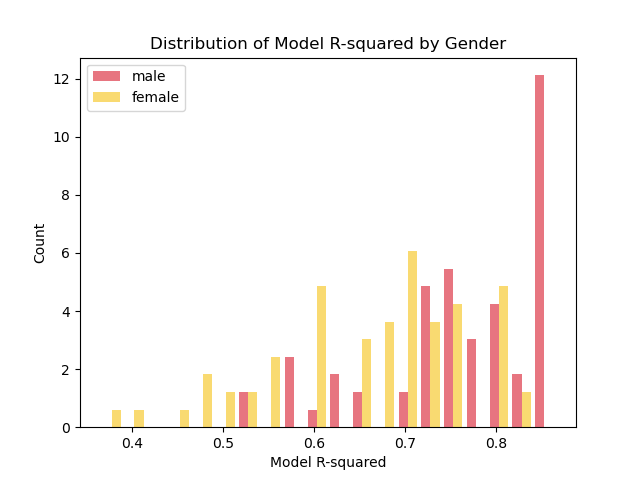
Future Directions
Our current research has indicated that AI models trained on female household data underperform models trained on male household data, yet there are many aspects left to explore. An important area for consideration is whether current household gender classification is appropriate, and, more broadly, whether future surveys can be improved to assess gender-specific wealth. Understanding what drives the differences between models trained on male and female data is also important - can other geospatial data features used in model training improve the performance of female models? Future research might also explore the possibility of utilizing wealth indices other than those produced by the DHS. The methods and code we have produced will hopefully provide an accessible approach for others to continue exploring these questions and others related to the role of gender in AI wealth estimation models.